As the fifth-born daughter to a poverty-stricken couple in a small village of Karnataka, Rinky would consider herself fortunate on days she wouldn’t have to sleep on an empty stomach. Her parents pressurised her to take care of her younger brother while they struggled to make ends meet. As the siblings grew up, the brother started going to a nearby school, while Rinky managed the household chores along with her sisters. A curious teenager, Rinky coerced her brother to teach her every now and then, including how to operate a smartphone they had recently acquired. When she turned 19, she mustered the courage to move to Bengaluru in search of a better life. She survived by doing menial jobs in the beginning such as cleaning houses, cooking and washing dishes. She earned a mere amount of Rs 15,000 (about 200 USD) every month, enough just to get by. She always felt disrespected having to deal with constant humiliation until someone in the neighbourhood advised her to learn driving and partner with the ride-hailing platform Ola.
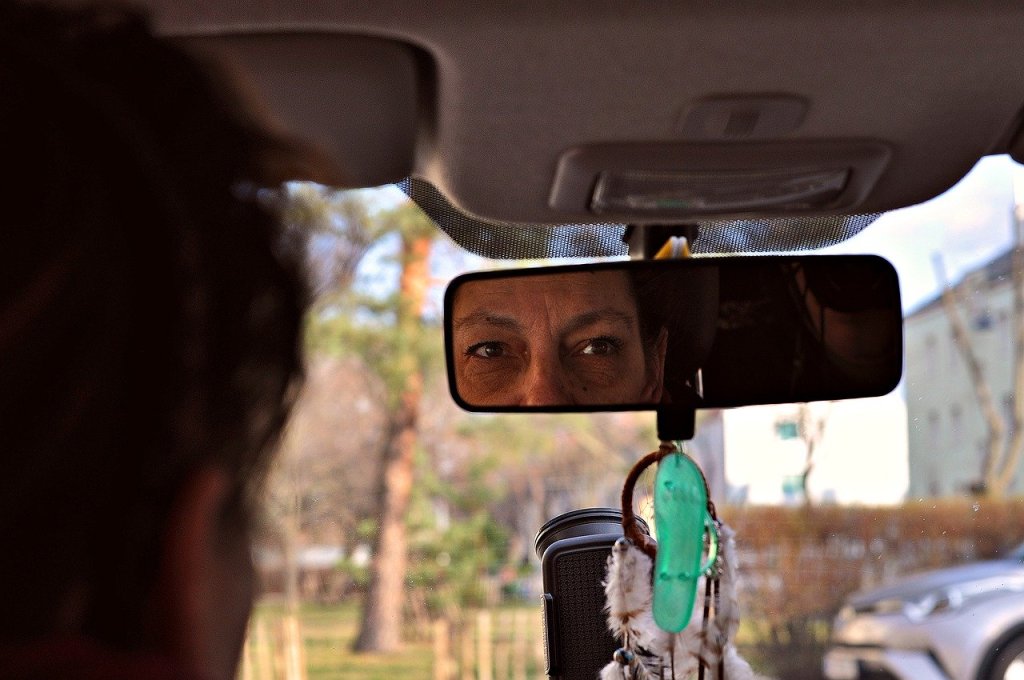
While there were initial hiccups in procuring the vehicle and learning how the app works, this move dramatically changed her life as it turned her into a micro-entrepreneur with a lucrative income of Rs 60,000 to take home every month. Besides allowing flexible work hours, the job provided her a sense of independence which was missing when she worked for others. She wasn’t really bothered about how the rides were assigned to her, though she always worried about her safety while boarding male passengers. At the same time, she was unable to comprehend how some of her colleagues earned more than her despite driving for similar number of hours.
“Rinky” is a composite character but represents stories of many such women for whom the platform-based economy has opened up a plethora of employment opportunities. The interesting aspect is that women workers are no longer confined to stereotypical jobs of salon or care workers; they are venturing into hitherto male domains such as cab driving and delivery services as well. The Babajob platform in fiscal 2016 recorded an increase of 153 per cent in women’s applications for driver jobs. According to the Road Transport Yearbook for 2015-16 (the latest such report available), 17.73 % of the 15 million licensed female drivers ride professionally. Though there are no distinct figures available for how many women are registered with Ola and Uber as drivers, ride-hailing app Ola confirmed a rise of 40 % every quarter in the number of female drivers with them. Moreover, cab aggregative service Uber announced to tie up with a Singapore-based company to train 50,000 women taxi drivers in India by this year.
Clearly, the ride-sharing economy is helping Indian women to break the shackles of patriarchy and improve their livelihood. However, the potential of these platforms cannot be fully utilized unless researchers turn an eye on the algorithms that govern them. These algorithms not only act as digital matchmakers assigning passengers to the drivers but regulate almost all aspects of the job – from monitoring workers’ behaviour to evaluating their performance. These machines often fail to treat workers as humans – as people who can fall sick, need a leisure break, socialise with others to stay motivated, de-route to pick up their kids from school, attend to an emergency at home, lose their temper occasionally, and moreover, coming to the work after facing physical abuse at home. In a normal work environment, employers tend to understand their team-members and often deal with compassion during tough times.

Research shows how these data-driven management systems especially in context of ride-sharing apps impact human workers negatively as they lack human-centred design. They discovered that sometimes female drivers did not accept male passengers without pictures at night only to be penalized by these algorithms later. Moreover, drivers complain of rude passengers, which is seldom taken into consideration by platform companies and it only lowers the driver’s acceptance rate and ratings.
Technology creators need to ask themselves how to ensure that algorithms are designed to enable workers and not just be optimized for customer satisfaction. Alternatively, they need to see the extension of worker’s satisfaction as that of customer gratification given these two realms reinforce one another. By sensitizing to the needs of women like Rinky who are perhaps stepping out in this male-dominated world for the first time, programmers could create a more empowering pathway for such women workers. With the entrenched gender norms burdening women with familial duties and limiting their access to education and skills training, the intervention by platform designers can promise genuine change. While cultural change often takes a long course, by placing women at the centre, designers can accelerate this shift.
More concretely, what if platform companies did the following:
They create a feedback/resolution system which accounts for rejections and safeguards ratings when women drivers reject certain passengers if they consider them as potential threat.
They can institute flexibility in terms of wanting to go home early and this shouldn’t be translated into ‘lower incentives’, after all this is the premise of gig economy.
AI should aim at promoting workers’ well-being, which means following a demanding or intensive piece of work (a long ride in this case), AI could recommend a relatively easier task for drivers.
Another aspect is to ensure transparency in terms of how the wages are allocated to different people and an understanding of how the autonomous systems impact ratings with also a system of redressal, i.e. one that allows for corrections etc.
Algorithms should encourage a community-building culture rather than individualism-oriented – social incentives could be given to those drivers who pick up rides when an assigned driver is unable to reach the destination instead of penalizing him or her.
While the in-built GPS system in apps can help drivers track public toilets and other places that could be used for restroom breaks, algorithms could be trained to adjust routes according to drivers’ needs and availability of amenities.
Moreover, popular ride-sharing platforms like Ola and Uber can consider assigning women passengers to women riders especially during the night-time. This move can make both the parties feel secure considering women dread boarding a taxi in an ‘unsafe’ country like India.
Across disciplines, if we brainstorm on reimagining these platforms as cooperative instead of competitive spaces, of human-centered versus optimization-centered, and as feminist-oriented and not just male-oriented, there may be more promise for our digital wellbeing.
——